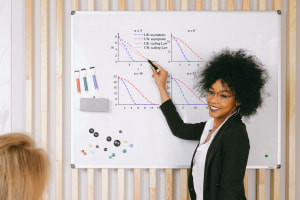
Lower Bounds Using Information Theory Tools
Lower Bounds Using Information Theory Tools
What You Will Learn In This Free Course
- Outline the concept of the informat...
- Describe the procedure for deriving...
- Discuss the relationship between Sh...
- Explain the process for deriving lo...
- Outline the concept of the information-theoretic lower bounds
- Describe the procedure for deriving lower bounds for compression problems
- Discuss the relationship between Shannon's source coding and ‘Shannon entropy’
- Explain the process for deriving lower bounds for random number generation
- Identify the methods of deriving minimax lower bound for statistical estimation
- Recognise the significance of empirical mean in deriving a lower bound
- Outline the concept of the minimax estimator
Complete This CPD Accredited Course & Get Your certificate!
Certify Your Skills
A CPD accredited Alison Diploma/Certificate certifies the skills you’ve learnedStand Out From The Crowd
Add your Alison Certification to your resumé and stay ahead of the competitionAdvance in Your Career
Share your Alison Certification with potential employers to show off your skills and capabilities
Learner Reviews & Feedback For Lower Bounds Using Information Theory Tools


Want to create a customised learning path for your team?
Our dedicated Learning Advisors are here to help you curate a customised learning path tailored to your organisation's needs and goals.
Not sure where to begin?
Discover the career most suitable for you and get started in the field with a step-by-step plan.
Get your PlanLearners Who Took This Course Also Enrolled In
- Quality Assurance (QA) - Techniques and Methodologies
- ISO Management System Audit Techniques and Best Practices
- Critical Facility: Critical Infrastructure
- Introduction to Conflict Management and Negotiation
- Human Resource Management - Discipline in Organizations
- Wind Energy – From Wind Turbines to Grid Integration
- Introduction to Pharmacy Practice
- Fundamentals Of Using Six Sigma In Supply Chains