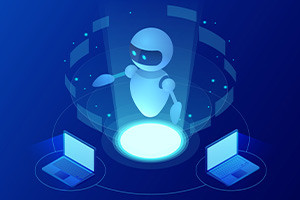
Diploma in Machine Learning with Python
Diploma in Machine Learning with Python
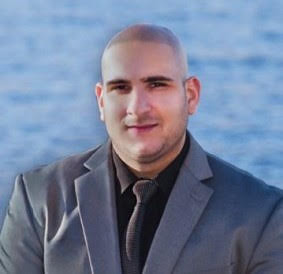
What You Will Learn In This Free Course
- Define machine learning...
- Explain feature scaling and data cl...
- Outline feature engineering...
- Distinguish between parametric and ...
- Define machine learning
- Explain feature scaling and data cleaning
- Outline feature engineering
- Distinguish between parametric and non-parametric models
- Discuss the exploratory data analysis on the Iris dataset
- Contrast categorical variables to numerical variables
- Explain decision trees and implementation
- Define ensemble learning
- Outline bootstrap sampling
- Compare SVM (support vector machines) hard margin to SVM soft margin
- List the various drawbacks of the PCA (principal component analysis)
- Recall the main applications of the PCA
Explore Careers Related To This Course
Complete This CPD Accredited Course & Get Your diploma!
Certify Your Skills
A CPD accredited Alison Diploma/Certificate certifies the skills youâve learnedStand Out From The Crowd
Add your Alison Certification to your resumé and stay ahead of the competitionAdvance in Your Career
Share your Alison Certification with potential employers to show off your skills and capabilities
Learner Reviews & Feedback For Diploma in Machine Learning with Python









Want to create a customised learning path for your team?
Our dedicated Learning Advisors are here to help you curate a customised learning path tailored to your organisation's needs and goals.
Not sure where to begin?
Discover the career most suitable for you and get started in the field with a step-by-step plan.
Get your PlanMore Free Online Courses by This Publisher
Learners Who Took This Course Also Enrolled In
- Machine Learning with Artificial Intelligence
- Diploma in Building High Accuracy Model With Core Machine Learning
- Diploma in Practical Machine Learning with Tensor Flow
- Artificial Intelligence and Machine Learning in Business
- Diploma in Python Programming
- Diploma in Cyber Security
- Machine Learning In Python Environment
- Building a Machine Learning App Interface