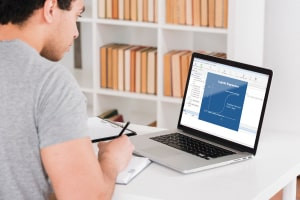
Logistic Regression in RStudio
This Free Online Course Includes:
- Hours of Learning
- CPD Accreditation
- Final Assessment
Logistic Regression in RStudio
In This Free Course, You Will Learn How To
- -
- -
- -
- -
Complete This CPD Accredited Course & Get Your Certificate!
Certify Your Skills
A CPD accredited Alison Diploma/Certificate certifies the skills you’ve learnedStand Out From The Crowd
Add your Alison Certification to your resumé and stay ahead of the competitionAdvance in Your Career
Share your Alison Certification with potential employers to show off your skills and capabilities
Learner Reviews & Feedback For Logistic Regression in RStudio
Want to create a customised learning path for your team?
Our dedicated Learning Advisors are here to help you curate a customised learning path tailored to your organisation's needs and goals.
Not sure where to begin?
Discover the career most suitable for you and get started in the field with a step-by-step plan.
Get your Plan