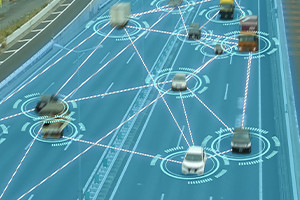
Computer Vision - Applications in Generative Models and Trends
- Learners already enrolled
This Free Online Course Includes:
- Hours of Learning
- CPD Accreditation
- Final Assessment
COURSE PUBLISHER -
Computer Vision - Applications in Generative Models and Trends
Learn about the ever-growing variants of generative adversarial networks and their uses with this free online course.
Did you know that there are ways to create realistic images based just on labelled sk... Did you know that there are ways to create realistic images based just on labelled sketches? This course illustrates the process of establishing substantial communication by mapping images between two domains for translating their content. Study the ways of tackling the failures of learning models using adversarial defence mechanisms and the process of ascertaining the optimal neural network design.
COURSE PUBLISHER -
In This Free Course, You Will Learn How To
- -
- -
- -
- -
Complete This CPD Accredited Course & Get Your Certificate!
Certify Your Skills
A CPD accredited Alison Diploma/Certificate certifies the skills you’ve learnedStand Out From The Crowd
Add your Alison Certification to your resumé and stay ahead of the competitionAdvance in Your Career
Share your Alison Certification with potential employers to show off your skills and capabilities
Learner Reviews & Feedback For Computer Vision - Applications in Generative Models and Trends
View More Reviews
Back to course information
Want to create a customised learning path for your team?
Our dedicated Learning Advisors are here to help you curate a customised learning path tailored to your organisation's needs and goals.